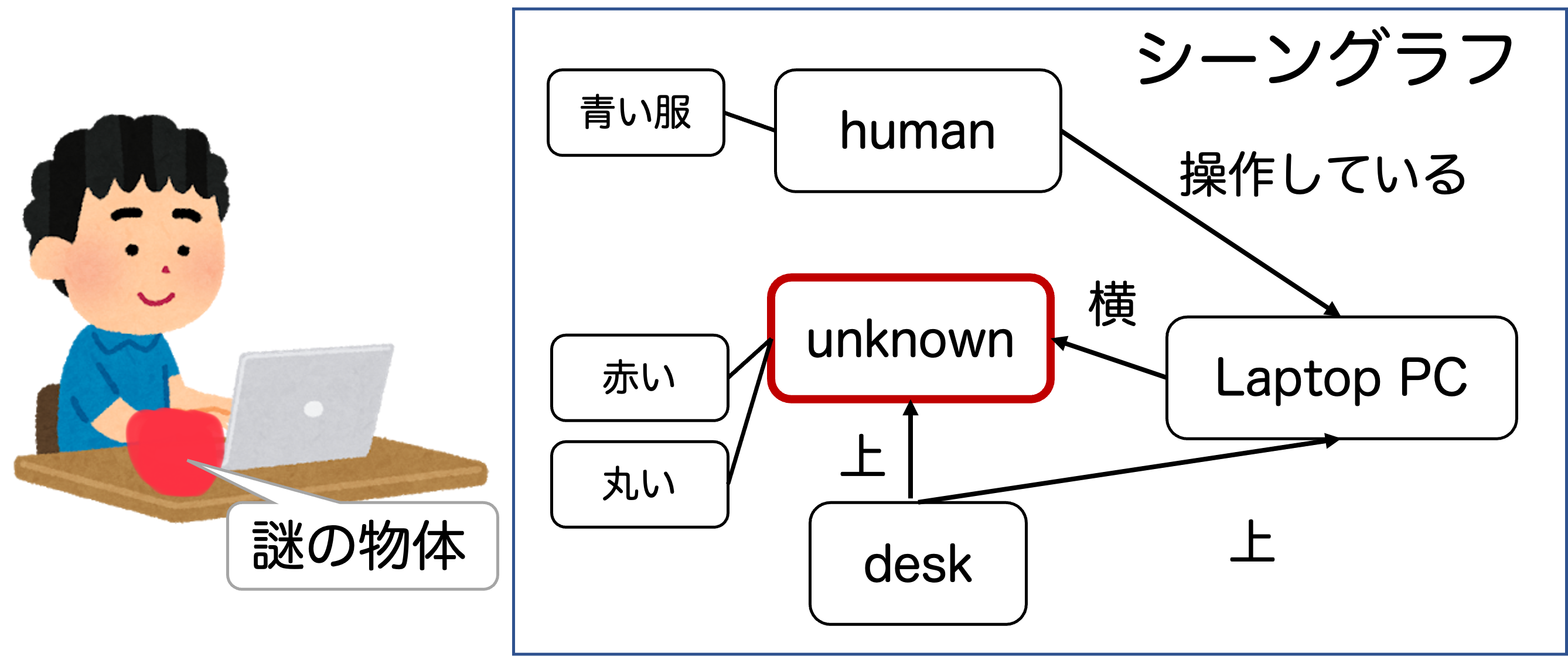
未知物体・未知事象の認識と記述
概要
人間は,知らない物体を見たとき,それが何かわからなくても何らかの物体であると認識できますが,ロボットは物体検出器が学習した物体しか検出できません.そこで,未学習の物体を未学習であると認識するOpen-set認識,及び新しい語彙で指定された対象を認識するOpen-vocabulary認識のタスクに取り組んでいます.また,物体だけでなく,行動など様々な未知事象に関する認識研究に対象を広げています.(この研究は日本学術振興会 科研費 基盤B 「拡張時空間シーングラフによる未知物体を含むシーン認識・記述基盤の構築」,科研費 基盤A 「能動的Open-world認識による知識拡張・環境認識基盤の構築」の支援を受けています.)
代表的な研究
- M. Sonogashira et al., Relationship-Aware Unknown Object Detection for Open-Set Scene Graph Generation, IEEE Access, 2024.
- T. T. Nguyen et al., One-stage open-vocabulary temporal action detection leveraging temporal multi-scale and action label features, FG2024.
- T. T. Nguyen et al., Zero-Shot Pill-Prescription Matching With Graph Convolutional Network and Contrastive Learning, IEEE Access, 2024.
- 薗頭ら,Open-setシーングラフ生成のための物体間の関係を考慮した未知物体検出, MIRU2023.
- M. Sonogashira & Y. Kawanishi, Towards Open-Set Scene Graph Generation with Unknown Objects, IEEE Access, 2022.
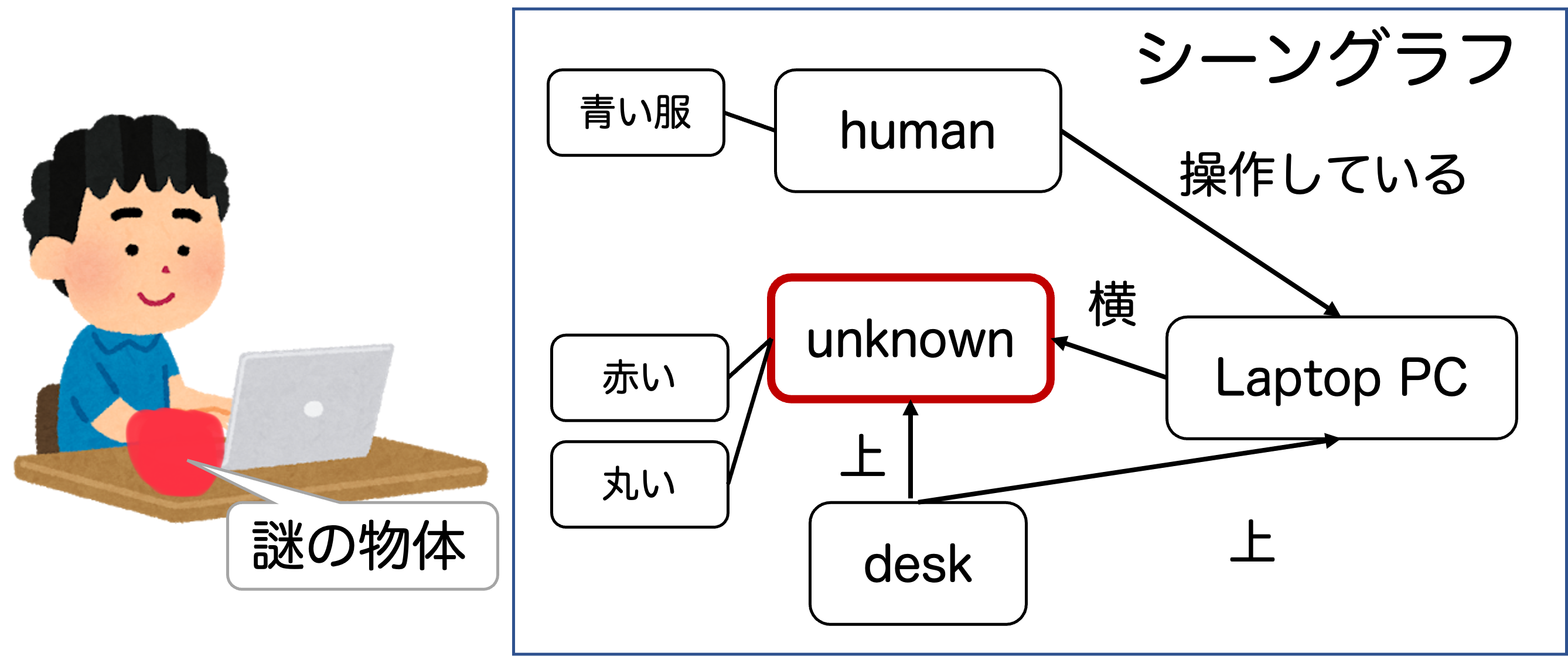
画像からのシーングラフ生成とその応用
概要
環境を高度に理解するため,物体の検出だけでなく,それらの物体同士の関係を推定・記述するシーングラフ生成というタスクに注目しています.また,それを応用し,複数の画像の内容を要約したり,キャプションを生成する研究に取り組んでいます.(この研究は日本学術振興会 科研費 基盤B 「拡張時空間シーングラフによる未知物体を含むシーン認識・記述基盤の構築」の支援を受けています.)
代表的な研究
- I. Phueaksri et al., Toward Visual Storytelling using Scene-Graph Contexts, MIRU2024, 2024.
- I. Phueaksri et al., Image-Collection Summarization using Scene-Graph Generation with External Knowledge, IEEE Access, 2024.
- I. Phueaksri et al., An Approach to Generate a Caption for an Image Collection using Scene Graph Generation, IEEE Access, 2023.
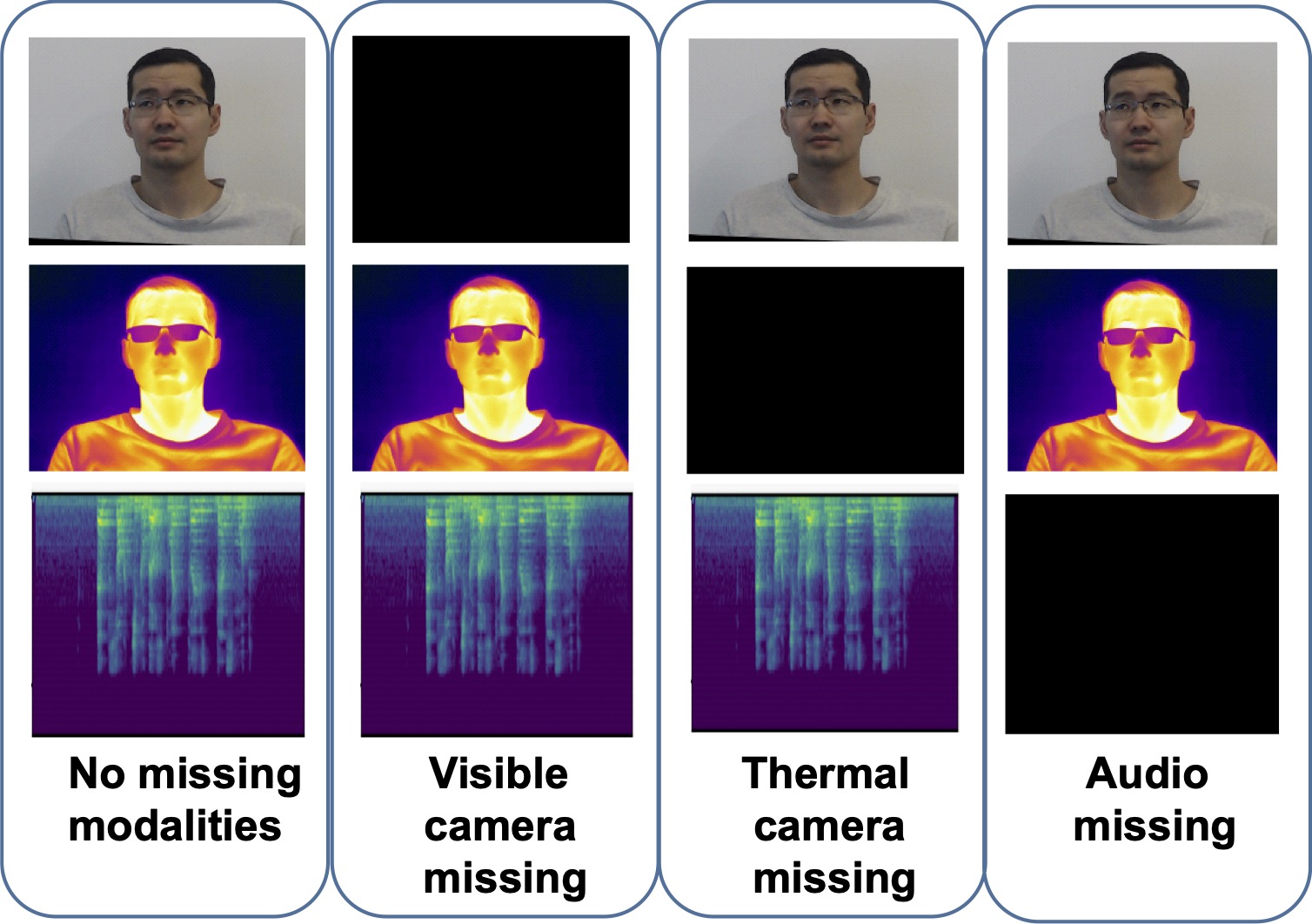
マルチモーダル情報を統合した認識基盤
概要
人間は目や耳を使って,映像や音声のモダリティをもとに周囲の情報を得ています.複数モダリティをもとに物事を認識する,マルチモーダル認識に関する研究をしています.特に,複数のモダリティが全て揃っていない状況での認識(モダリティ欠損)の問題に取り組んでいます.
代表的な研究
- V. John and Y. Kawanishi, Progressive Learning of a Multimodal Classifier Accounting for Different Modality Combinationsi, Sensors, 2023.
- V. John and Y. Kawanishi, A Multimodal Sensor Fusion Framework Robust to Missing Modalities for Person Recognition, arxiv:2210.10972, 2022.
- V. John and Y. Kawanishi, Combining Knowledge Distillation and Transfer Learning for Sensor Fusion in Visible and Thermal Camera-based Person Classification, MVA2023.
- V. John and Y. Kawanishi, Multimodal Cascaded Framework with Metric Learning Robust to Missing Modalities for Person Classification, ACM MMSys', 2023.
- V. John and Y. Kawanishi, Audio-Visual Sensor Fusion Framework using Person Attributes Robust to Missing Visual Modality for Person Recognition, MMM2023.
- V. John and Y. Kawanishi, A Multimodal Sensor Fusion Framework Robust to Missing Modalities for Person Recognition, ACM MM Asia 2022.
- V. John and Y. Kawanishi, Audio and Video-Based Emotion Recognition Using Multimodal Transformers, ICPR2022.
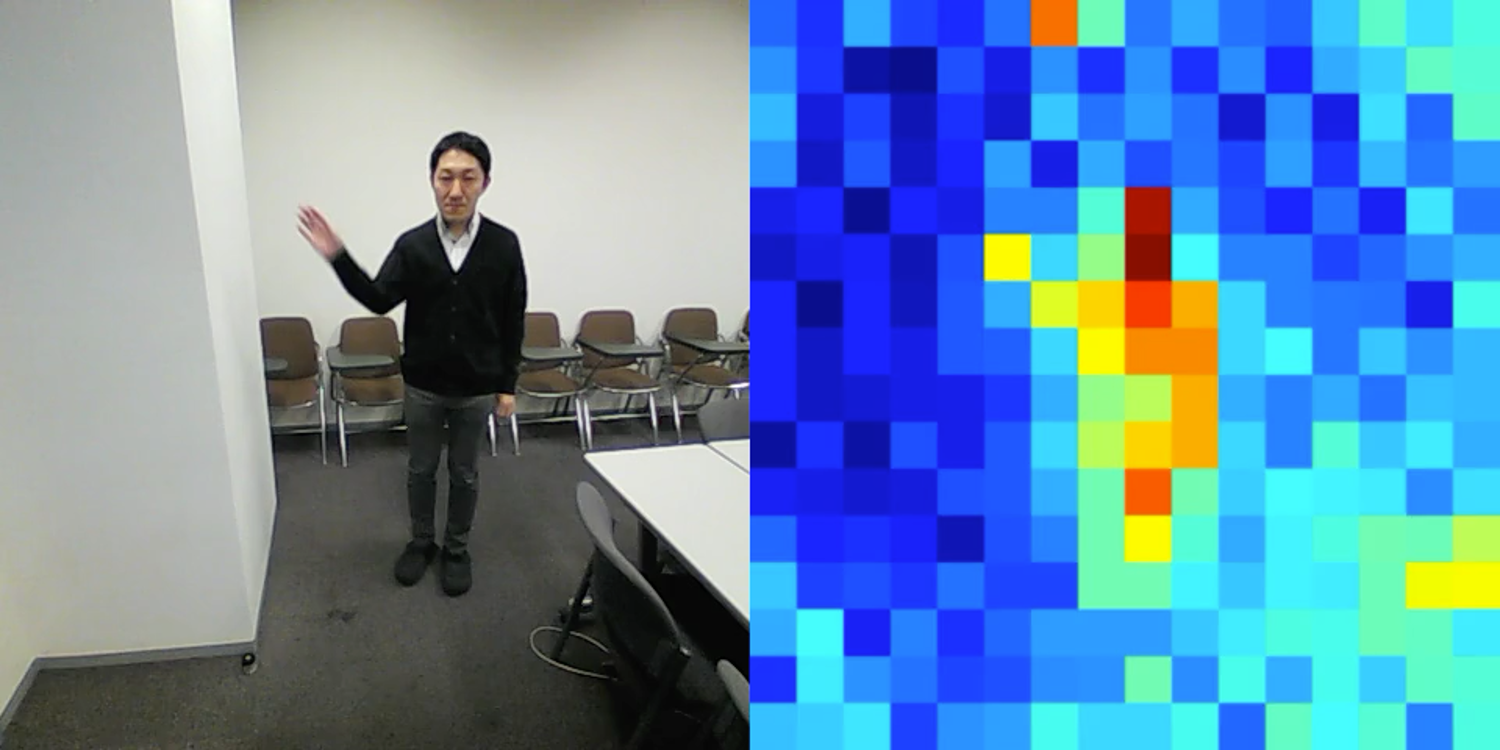
超低解像度遠赤外線画像の認識
概要
高齢者の見守りのためには,プライバシに考慮し,また夜間でも認識可能なセンシングが必要です.そこで,超低解像度な遠赤外線画像に着目しています.超低解像度であるためにプライバシは問題にならず,遠赤外線を捉えることで夜間でもセンシングが出来ます.これを用いて,人物の行動認識や姿勢推定に関する研究をしています.
代表的な研究
- S. Iwata et al., LFIR2Pose: Pose Estimation from an Extremely Low-Resolution FIR Image Sequence, ICPR2020.
- Y. Kawanishi et al., Voting-based Hand-Waving Gesture Spotting from a Low-Resolution Far-Infrared Image Sequence, VCIP2018.
- T. Kawashima et al., Action Recognition from Extremely Low-Resolution Thermal Image Sequence, AVSS2017.
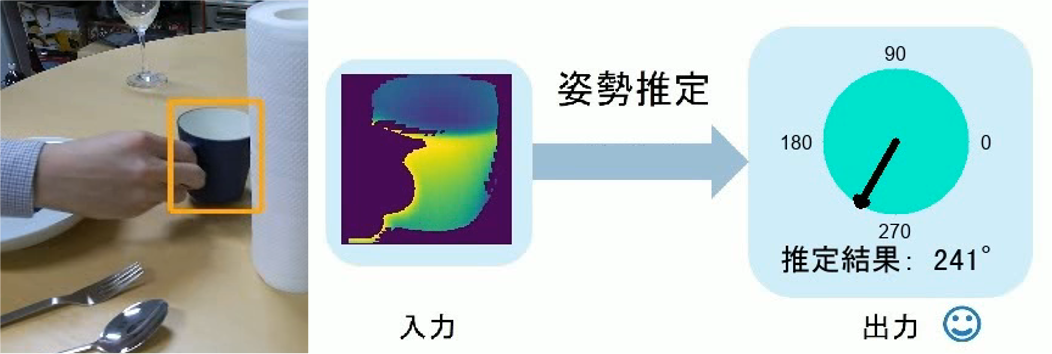
距離画像を用いた物体姿勢推定
概要
ロボットが物体を掴んだり操作したりする際には,物体の姿勢を正しく認識する必要があります.最近は距離画像センサが多くのロボットに搭載されるようになって来ています.そこで,距離画像を用いて物体の姿勢を推定する研究に取り組んでいます.
代表的な研究
- H. Tatemichi et al., Category-level Object Pose Estimation in Heavily Cluttered Scenes by Generalized Two-stage Shape Reconstructor, IEEE Access, 2024.
- N. M. Z. Hashim et al., Best Next-Viewpoint Recommendation by Selecting Minimum Pose Ambiguity for Category-level Object Pose Estimation, JSPE, 2021.
- H. Tatemichi et al., Median-Shape Representation Learning for Category-Level Object Pose Estimation in Cluttered Environments, ICPR2020.
- H. Ninomiya et al., Deep Manifold Embedding for 3D Object Pose Estimation, VISAPP2017.
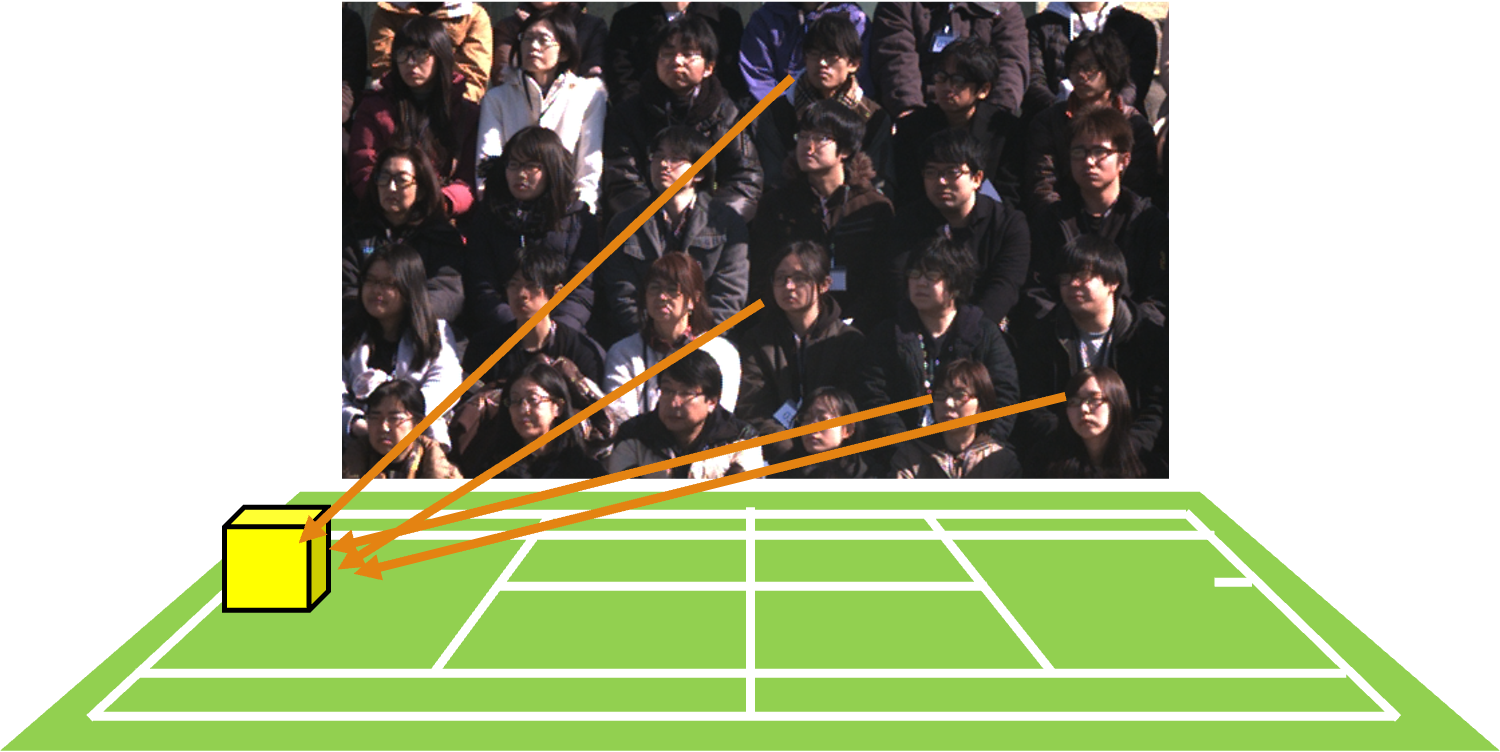
群衆の視線推定
概要
多数の人物が,何に注目しているのかを知ることは,スポーツ観戦者や音楽ライブイベント参加者の興味の推定に有用です.そこで,多数の人物を同時に写した映像から,そこに写っている人物の多くが,何に注目しているのかを推定する研究に取り組んでいます.
代表的な研究
- 武田ら, 観衆の顔向きの時空間統合による注目対象の位置及び被注目度の推定, 電子情報通信学会論文誌A, 2023.
- Y. Kodama et al., Localizing the Gaze Target of a Crowd of People, ACCV2018 Workshop.
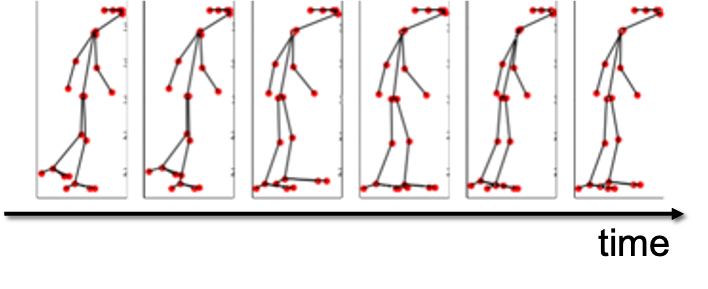
骨格系列の変化による行動認識・予測
概要
人の骨格がどのように変化しているかに着目することで,その人が何をしているのか,どういう状態なのかを知ることが出来ます.最近,画像から人物の骨格を精度よく推定できる技術が出てきたことから,こうした技術を用いて推定した骨格を,様々な認識や予測に応用する研究に取り組んでいます.特に,見た目からだけでは判断しづらい,荷物の重さや,手荷物所持者がどのくらい負担に感じているか,等を認識することにも取り組んでいます.
代表的な研究
- M. Mizuno et al., Subjective Baggage-Weight Estimation based on Human Walking Behavior, IEEE Access, 2024.
- M. Mizuno et al., Subjective Baggage-Weight Estimation from Gait ---Can you estimate how heavy the person feels?---, VISAPP2023.
- T. Fujita, Future Pose Prediction from 3D Human Skeleton Sequence with Surrounding Situation, Sensors, 2023.
- T. Fujita et al., Human Pose Prediction by Progressive Generation in Multi-scale Frequency Domain, MVA2023.
- T. Fujita et al., Toward Surroundings-aware Temporal Prediction of 3D Human Skeleton Sequence, ICPR2022 Workshop (T-CAP).
- 藤田ら, 人物周辺情報を活用した3次元骨格の時系列予測, MIRU2022.
- 水野ら, 歩容からの身体特徴と動作特徴の分離による手荷物の物理的・主観的重さ推定, MIRU2022.
- 水野ら, 個人差を考慮した歩き方からの手荷物の重さ推定の検討, 電子情報通信学会技術研究報告(PRMU), PRMU2021-56, 2021/12/17
- N. Nishida et al., SOANets: Encoder-Decoder based Skeleton Orientation Alignment Network for White Cane User Recognition from 2D Human Skeleton Sequence, VISAPP2020.
- O. Temuroglu et al, Occlusion-Aware Skeleton Trajectory Representation for Abnormal Behavior Detection, IW-FCV2020.
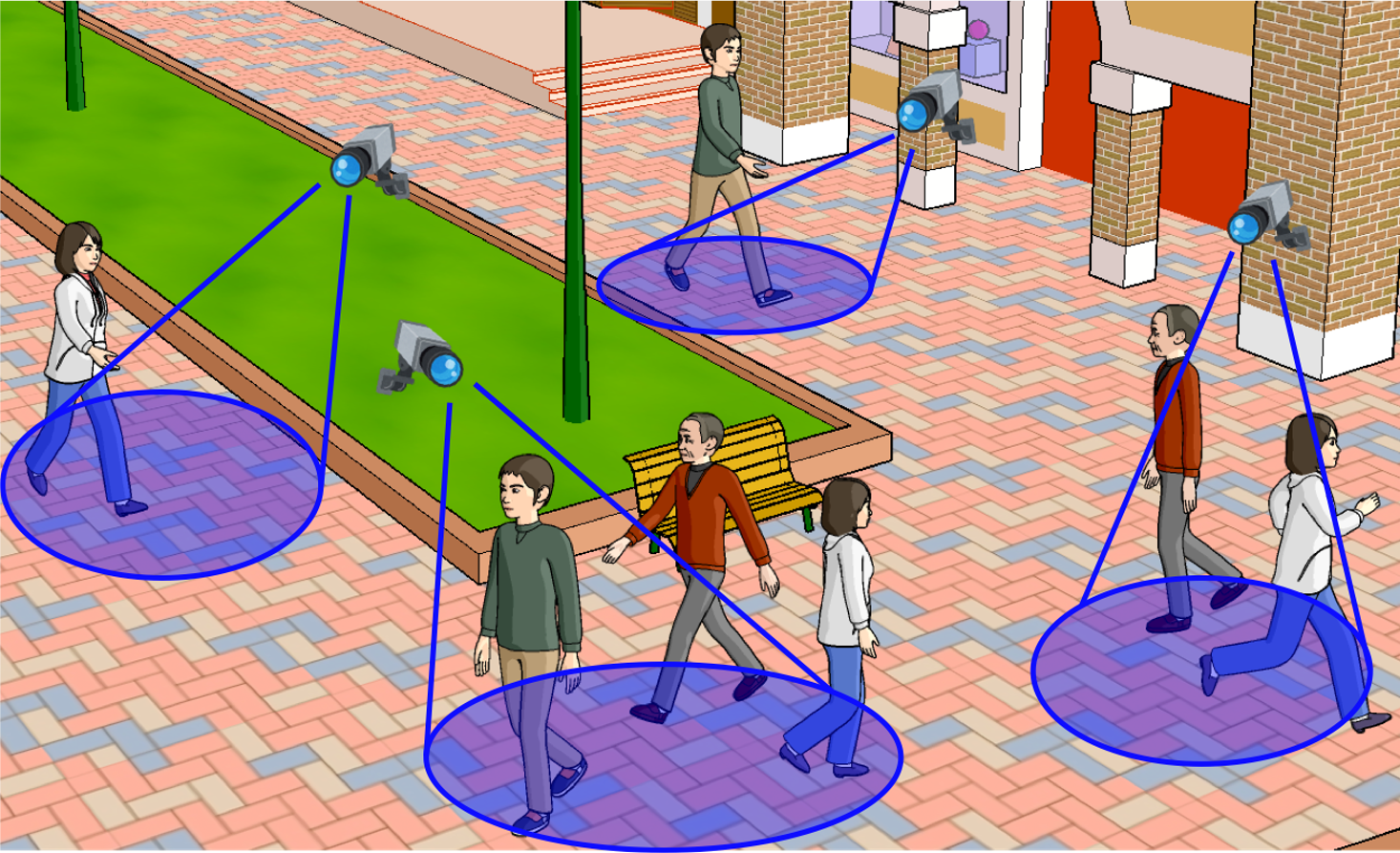
広範囲の複数人物追跡
概要
広範囲にわたる人物の移動軌跡を知ることは,人流解析や迷子探し等の技術において重要です.複数のカメラによって広範囲を観測する状況で,どの人がどこを通ってどこへ行ったかを知るために,各カメラ視野内での人物追跡と,カメラ視野間での人物の対応付けに関する研究に取り組んでいます.
代表的な研究
- Y. Kawanishi, Label-Based Multiple Object Ensemble Tracking with Randomized Frame Dropping, ICPR2022.
- Y. Kawanishi et al., Trajectory Ensemble: Multiple Persons Consensus Tracking across Non-overlapping Multiple Cameras over Randomly Dropped Camera Networks, CVPR2017 Workshop.
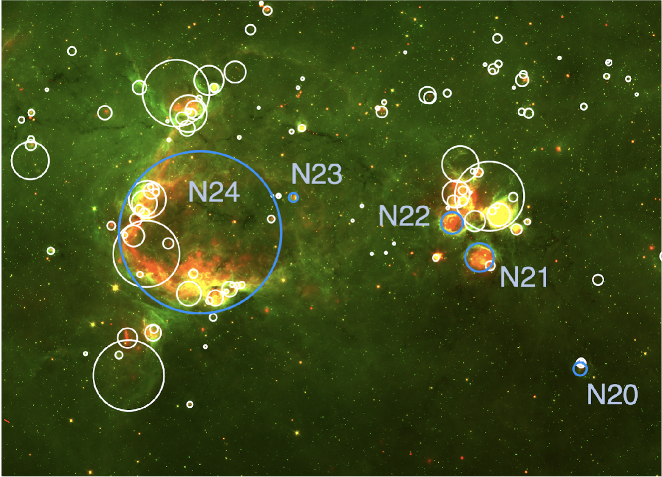
画像認識技術の他分野への応用
概要
画像認識技術は,生命科学や天文学など,様々な分野でも期待されています.我々も,天文学や考古学の分野に画像認識技術を応用した研究に取り組んでいます.例えば,星が形成されつつある領域の検出であったり,発掘した土器の産地同定などに取り組んでいます.
代表的な研究
- Y. Kawae, et al., 3D Survey of the Menkaure Pyramid, Virtual Annual Meeting, American Research Center in Egypt, 2024.
- Y. Shimajiri et al., Predicting reliable H2 column density maps from molecular line data using machine learning, MNRAS, 2023.
- S. Fujita et al., Distance determination of molecular clouds in the first quadrant of the Galactic plane using deep learning, Protostars and Planets VII, 2023.
- S. Fujita et al., Distance determination of molecular clouds in the first quadrant of the Galactic plane using deep learning: I. Method and results, ASJ, 2023.
- S. Nishimoto et al., Development of a high-speed identification model for infrared-ring structures using deep learningi, SPIE Astronomical Telescopes + Instrumentation 2022.
- 堀ら, 埋蔵文化財保護行政における3Dデータ活用 ―機械学習を活用した須恵器杯蓋の産地同定手法―, 情報文化学会誌, 2022.
- 野原ら, 3Dデータを活用した埋蔵文化財業務の効率化 ~実測図自動作成手法の提案~, 社会情報学会中部支部・研究発表論文集, 2021.
- 井上ら, 人工知能による機械学習を用いた須恵器資料の断面形状分析
日本情報考古学会第43回講演論文集, 2020. - S. Ueda et al., Identification of infrared-ring structures by convolutional neural network, SPIE Astronomical Telescopes + Instrumentation 2020